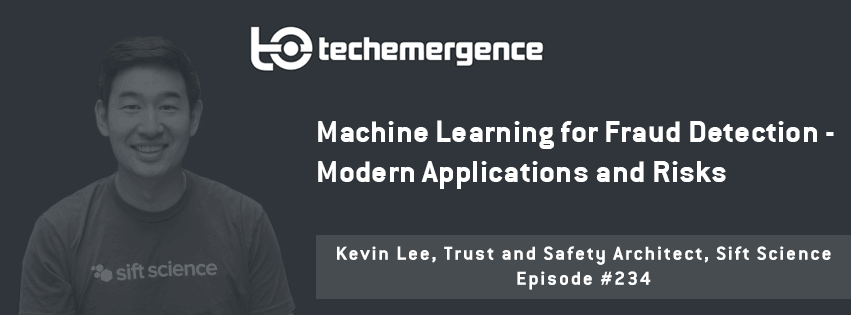
Episode Summary1: Fraud attacks have become much more sophisticated. Account takeovers are happening more often. Many security attacks involve multiple methods and unexpected attacks can devastate businesses in just a few days, as we saw with Neiman Marcus and Target. False promotion and abuse is seen not only on social media sites but is also targeted at business. To combat these risks, fraud solutions need to be smarter to keep pace with fraudsters to prevent attacks and react quickly when they do happen. This requires a fast-learning solution with the ability to continually evolve – which calls for the application machine learning for fraud detection. In this episode we talk to Kevin Lee from Sift Science and examine the shifts in the info security landscape over the past ten or fifteen year. Lee also highlights what new kinds of fraud are now possible and what machine learning solutions are available.
Guest: Kevin Lee
Expertise: Fraud assessment and security, risk management.
Brief Recognition: Kevin Lee is the resident Trust and Safety Architect at Sift Science, a global fraud detection system which uses machine learning technology to predict fraudulent behavior. He has previously managed risk and safety organizations at Facebook, Square and Google
Big Idea:
Modern fraud has to do with risk and trust, not just with payments – any platform for content, community, or commerce has fraud risk of some kind
Today’s fraud risks extend far beyond payment fraud. Now companies have to protect themselves and their information against identity theft, degradation of trust, fake user accounts, as well as other safety issues. This means that fraud detection must get smarter. Developing and implementing a fraud risk management plan can be a challenge. Once inherent fraud risks have been identified, it’s not enough just to apply prevention controls. But if you can predict fraudulent behavior, you can stay one step ahead of the game.
Large-scale machine learning technology can collect huge amounts of data relating to fraudulent activities worldwide and analyze it instantly. Thousands of traces left behind by fraudsters, which might otherwise have remained unconnected in the vast ocean of data, are now linked to produce a clear predictor of fraud threats. As the AI crunches through all this data, it is able to detect anomalies and indicate probable incidences of abuse. Companies like Yelp, Airbnb and Jet.com already use these insights to protect themselves from content and promo abuse, payment fraud, fake accounts and account takeover. Whatever your industry, as a business owner, this type of AI solution can be of benefit.
(We cover credit card payment fraud and some examples of account fraud in our full article on “Everyday Examples of AI”, which may be of interest to readers of this interview article.)
Turning Insight to Action: Fraud is a threat to every industry. As a business leader, you want to feel secure that your company’s vulnerability to fraud is minimized. What are your company’s biggest security concerns? What are some of the fraud schemes and strategies that your business is vulnerable to and how will they change in the near future? Using machine learning to analyze data and discover patterns is nothing new, but recent developments in technology has led to differences in how machine learning algorithms have evolved and are being applied. One of these applications is fraud prevention, where it can be used to sift through large amounts of data, develop risk patterns and statistics and highlight a business’s weaknesses.
By using machine learning to identifying your company’s own biggest fraud risks and predict and guard against those risks, you can protect your company, your clients and your reputation, while cutting operational costs and increasing user confidence.
Interview Highlights on Machine Learning for Fraud Detection:
The following is a condensed version of the full audio interview, which is available in the above links on Emerj’s SoundCloud and iTunes stations.
(3.35) Is there a difference between where fraud detection and technology is being used now as opposed to where fraud detection was being applied five or ten years ago?
Kevin Lee: Ten or fifteen years ago mainly people were concerned about payment fraud or credit card fraud online…It has become a bit more pervasive, where the issue is not just about the credit card, it’s about identities and even the way people interact online. Before it used to be “oh I want to buy a laptop, I’m just going to go to a website, go through the guest checkout and I’m done, but now people are moving more and more of their psyches online…And what has become a hot topic lately is account takeover, identity theft. Now eCommerce has come up…originally eBay was a master merchant but then they enabled people to sell stuff from their garage and that introduced a whole new variable where before, if you were a merchant, you obviously had confidence in yourself, of course I’m going to deliver these goods that I’m selling, but now you get into a scenario where I could be bad, the merchant could be bad or both could be bad and so it becomes more complex to figure out who’s bad, what’s the story and what is going on in this space. As a result it’s become much more difficult to decipher who’s good and who’s not.
(7.17) Where’s the shift in focus given all the new factors at play?
Kevin Lee: Five to ten years ago, the main way fraud was perpetrated online was by creating a fake account, maybe use a stolen credit card and make a transaction, that was the main issue. Now what’s happening, partly because of data breached that are occurring, another reason is that people are just moving around more of their identities online, account takeover is becoming the next thing…Many vendors out there have done a very good job of spotting and killing fake accounts and so the fraudsters and spammers out there, they’re running a business as well, so if they’re not getting their return on investment, by creating these fake accounts, they need to go elsewhere…The next step is around compromising identities and accounts…we are seeing much more targeted and specific hacking…The incidences may be less, but the damage per incidence is much more.
(12.51) Would we be using a similar kind of technology for these different types of fraud, for example would we use the same for transaction fraud, Facebook use or password hacking? Do we put those in the same bucket of “machine learning for fraud detection” here?
Kevin Lee: It can vary per company a bit but really there are two main buckets, there’s the data security side of it, in terms of theft of credit cards are they hashed, or encrypted and then there’s the info security in terms of risk and abuse of payment, promotion or content… trust and safety and risk goes beyond just financial.
Fraud now it sounds like extends to something like someone on Yelp doing fake reviews, or someone with a blogging service with some really spammy, scraped together, re-hashed articles that are going all over the place…for these example we could use the term fraudulent content, which clearly will have, at a certain scale, a negative financial impact on a company. Am I putting the right color on this in terms of what this type of fraud might be?
Kevin Lee: Yes. It can be more difficult to measure. With transactions if you have a chargeback you have some kind of ground truth, but when it comes to these types of fraud it can have an effect on a company in terms of lifetime value.
(16.29) I guess companies now are more aware of this type of abuse and the potential financial implications…If I’m detecting a fraudulent charge, if I’m detecting an unusual eBay customer profile, if I’m detecting some unusual review behaviors on Yelp, whether this is a market place and it’s content, whether it’s engagement, whether it’s a transaction…these are all examples of I guess what we could call anomaly detection. Companies like yours…have some understanding of what normalcy looks like for a customer…and they have a sense for when he or she’s not doing something right. Would these all be examples of anomaly detection?
Kevin Lee: Definitely. And this is really where machine learning comes in… A human analyst or a human reviewer can only look at a handful of signals at a time and make a determination. But there is enough data out there and that’s really when machine learning comes into play. Because it’s literally able to crunch thousands of signals and look at probabilities of abuse or probabilities or fraud. That’s really where the industry is going from a machine learning viewpoint.
Related “AI in Industry” Interviews on Fraud and Data Security:
At Emerj our goal is the provide business leaders with insights and facts about the applications and implications of artificial intelligence. Security and fraud are a sectors of wide interest in the business community – as they effect essentially all industries. Our AI in Industry podcast (iTunes) is the easiest way to stay up to date with our latest security interviews – here’s a selection of related interviews that might be of interest to readers of this article:
- Current Challenges and Future Applications of Machine Learning in Infosecurity – with Uday Veeramachaneni, CEO of Patternex
- Malicious AI and the Dark Side of Data Security – with Justin Feir, Director of Cyber Intelligence at Darktrace
- Network Intrusion Detection Using Machine Learning – with Sal Stolfo, SEO of Allure Security